Artificial Intelligence has advantages. It can analyze large amounts of data in a short time. It can work without fatigue or boredom to process data. It can generate diverse and valuable insights from datasets. Artificial Intelligence is well-suited for large and complex data settings.
Generative AI
At Quantum Insights we leverage the power of Generative AI to create innovative solutions for our clients. We believe that Generative AI is a game-changing technology that enables to generate new and exciting content, such as text, images, audio, video, and code, from data or prompts.
Generative AI is a type of artificial intelligence (AI) system that learns the patterns and structure of its input training data, and then generates new data that has similar characteristics. Generative AI can foster innovation and creativity, as well as optimize business processes and workflows.
At Quantum Insights, we use Generative AI to:
- Enhance the design and development of products, services, software, and applications.
- Support the analysis and synthesis of data, information, and knowledge.
- Enable the personalization and customization of user experiences and interactions.
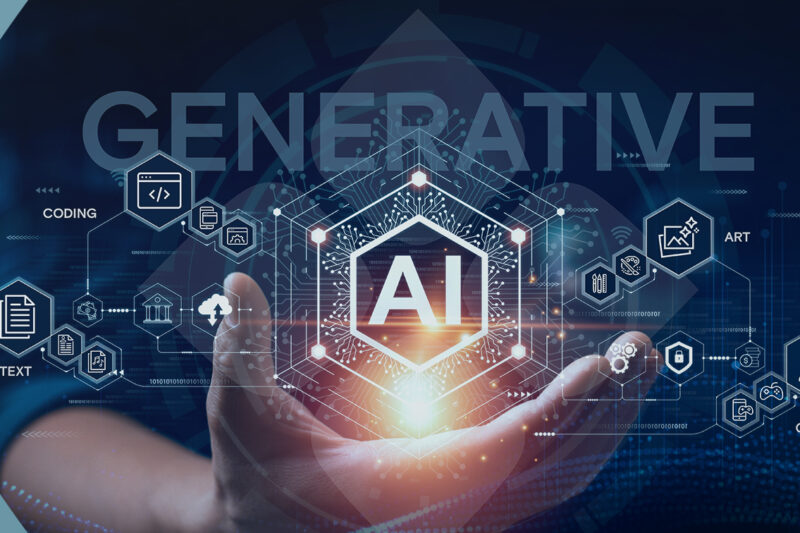
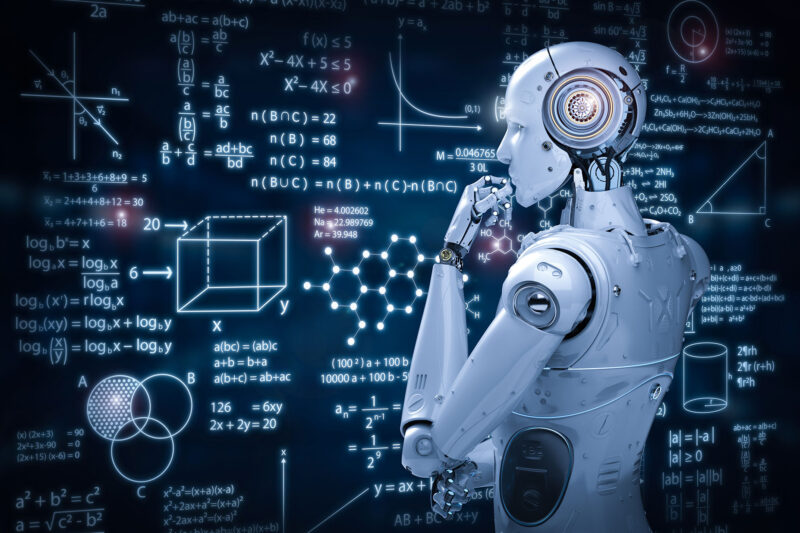
Machine Learning
Applications that provide systems the ability to automatically learn and improve from experience without being explicitly programmed to perform specific tasks.
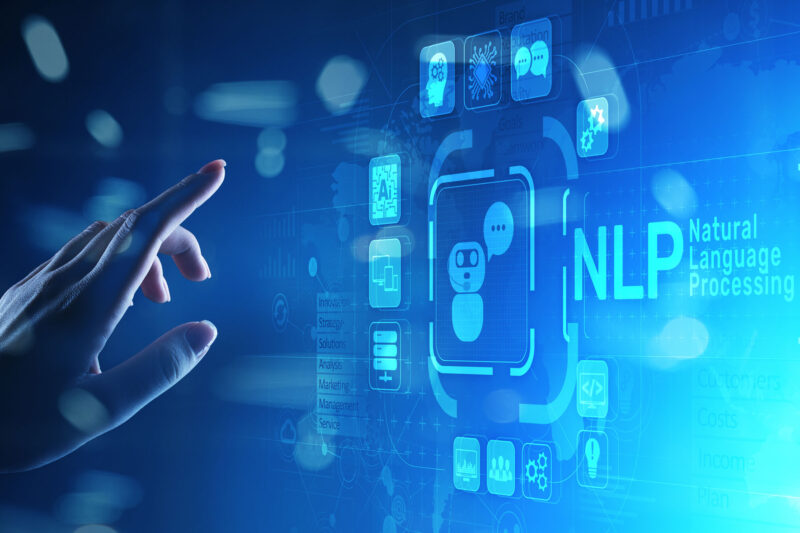
Natural Language Processing
Applications that read, decipher, understand, and make sense of the human language, to assist in the interaction between computers and humans.
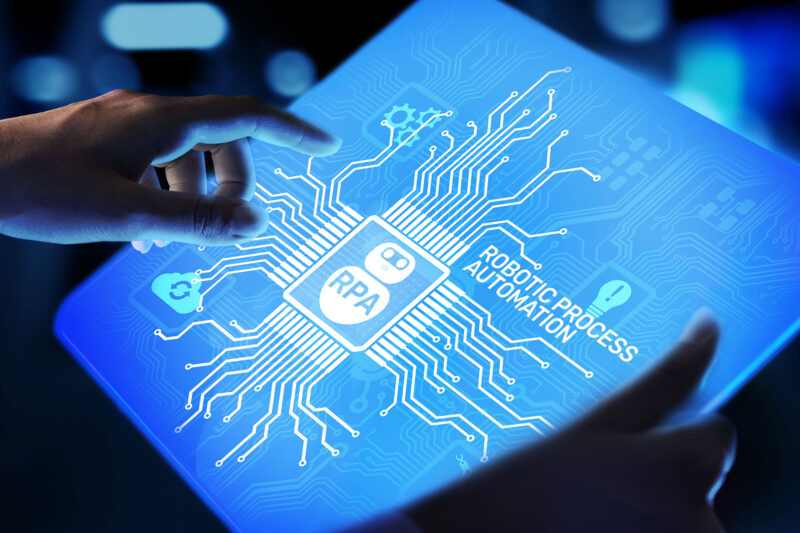
Robotic Process Automation
Applications and tools that partially or fully automate human activities that are manual, rule-based, and/or repetitive.
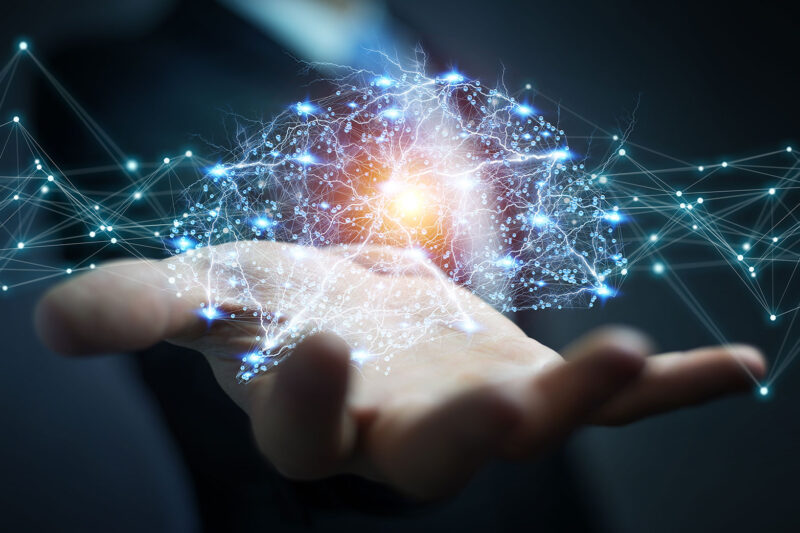
Neural Networks
Systems that are built using the inspiration of biological neural networks to learn to perform a breadth of tasks by considering examples